Author: Bernhard Steindl
Supervisor: Julia Neidhardt, Co-Supervisor: Mete Sertkan
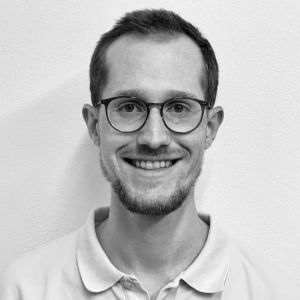
Abstract
Recommender systems have become an essential part of our modern lives. Recommendation algorithms support users by recommending items tailored to their interests and by assisting in discovering new items. For example, some online news platforms use recommendation models to suggest news articles to users based on their news consumption.
However, recommender systems also pose risks, such that certain user groups may be disadvantaged concerning the utility of recommendations. Users might get trapped in filter bubbles by biased recommendations that primarily reflect existing interests and opinions.
Excessive personalization of recommendations may result in a lack of content diversity, making it difficult for users to find a balanced range of viewpoints.
My thesis addresses the problem that some user groups may be treated unfairly in terms of the recommendations they receive.
The work aims to study the recommendation fairness among user communities detected in a social network built from user interaction data in terms of accuracy and beyond-accuracy measures. Communities in social networks can be groups of users who share common interests or activities. From this thesis, we expect to gain insights into the fairness of recommendations when using different recommendation algorithms to alleviate the disparate treatment of user groups.
Data from the Austrian online news platform “DER STANDARD” is used for this study.