Author: Boris Staykov
Supervisor: Julia Neidhardt, Co-Supervisor: Thomas E. Kolb
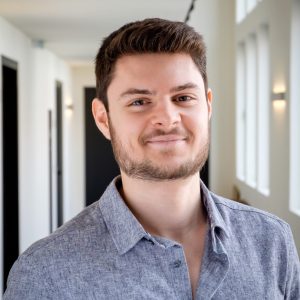
Abstract
Recommender systems play a pivotal role in personalizing user experiences across various domains such as e-commerce, news, and entertainment platforms. However, the presence of bias within these systems poses significant challenges, potentially leading to unfair treatment of users. This thesis addresses the critical issue of bias in recommender systems, focusing specifically on the dynamic nature of bias over time. Through the analysis of anonymized user behavior data from a popular Austrian newspaper website, the research aims to detect, measure, store, and visualize different types of bias, e.g. popularity bias.
The proposed framework involves employing advanced data analytics techniques and a web-based visualization tool to provide insights into bias dynamics. The study utilizes a Design Science Research methodology to iteratively develop and evaluate the proposed solution, ensuring its relevance, rigor, and design effectiveness. Moreover, privacy and ethical considerations are paramount, with a commitment to handling anonymized data responsibly and respecting user privacy.
The research contributes to both academia and industry by advancing understanding of bias in recommender systems and providing practical tools for bias detection and visualization. Ultimately, the thesis seeks to enhance the fairness and transparency of recommender systems, promoting a more equitable user experience for all.